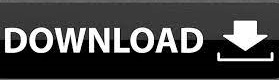
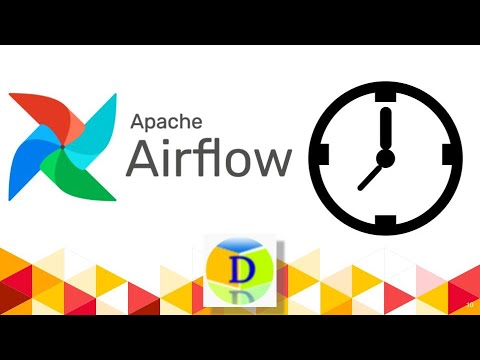
However, when the DAG is being automatically scheduled, with certain Logical is because of the abstract nature of it having multiple meanings,ĭepending on the context of the DAG run itself.įor example, if a DAG run is manually triggered by the user, its logical date would be theĭate and time of which the DAG run was triggered, and the value should be equal (formally known as execution date), which describes the intended time aĭAG run is scheduled or triggered. Run’s start and end date, there is another date called logical date This period describes the time when the DAG actually ‘ran.’ Aside from the DAG Tasks specified inside a DAG are also instantiated intoĪ DAG run will have a start date when it starts, and end date when it ends. In much the same way a DAG instantiates into a DAG Run every time it’s run, Run will have one data interval covering a single day in that 3 month period,Īnd that data interval is all the tasks, operators and sensors inside the DAG Those DAG Runs will all have been started on the same actual day, but each DAG The previous 3 months of data-no problem, since Airflow can backfill the DAGĪnd run copies of it for every day in those previous 3 months, all at once. It’s been rewritten, and you want to run it on

Same DAG, and each has a defined data interval, which identifies the period ofĪs an example of why this is useful, consider writing a DAG that processes aĭaily set of experimental data. If schedule is not enough to express the DAG’s schedule, see Timetables.įor more information on logical date, see Data Interval andĮvery time you run a DAG, you are creating a new instance of that DAG whichĪirflow calls a DAG Run. The SQLite database and default configuration for your Airflow deployment are initialized in the airflow directory.For more information on schedule values, see DAG Run. In a production Airflow deployment, you would configure Airflow with a standard database. Initialize a SQLite database that Airflow uses to track metadata. Airflow uses the dags directory to store DAG definitions.
#Trigger airflow dag via api install#
Install Airflow and the Airflow Databricks provider packages.Ĭreate an airflow/dags directory. Initialize an environment variable named AIRFLOW_HOME set to the path of the airflow directory.
#Trigger airflow dag via api code#
This isolation helps reduce unexpected package version mismatches and code dependency collisions. Databricks recommends using a Python virtual environment to isolate package versions and code dependencies to that environment. Use pipenv to create and spawn a Python virtual environment. Pipenv install apache-airflow-providers-databricksĪirflow users create -username admin -firstname -lastname -role Admin -email you copy and run the script above, you perform these steps:Ĭreate a directory named airflow and change into that directory.

Run tasks conditionally in a Databricks job.Pass context about job runs into job tasks.Share information between tasks in a Databricks job.
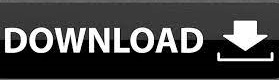